#E0053 Prediction and predictor elucidation of metabolic syndrome onset among young workers using machine learning techniques: A nationwide study in Japan
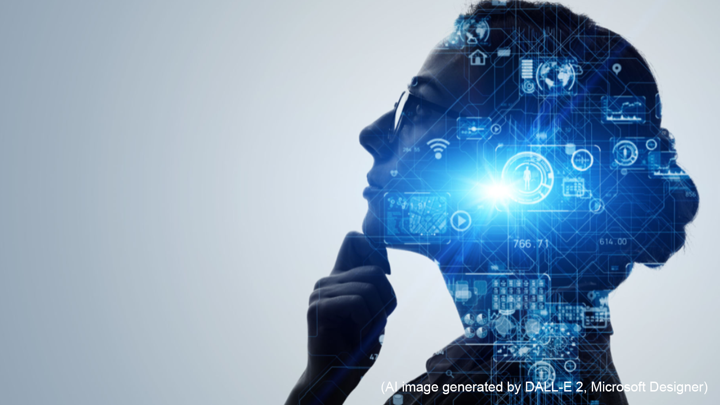
Machine Learning Models Predict Onset of Metabolic Syndrome among Japanese Workers
Metabolic syndrome (MS) refers to a group of metabolic disorders that predisposes the sufferer to chronic, debilitating conditions such as diabetes and cardiovascular disease. Given the high worldwide prevalence of MS and its emerging influence on health economics, occupation, and inequalities, measures for preventing the onset of MS are imperative. To this end, previous research suggests that identification of high-risk individuals in their 30s could be useful.
In Japan, preventive health guidance against MS is currently provided to people aged 40 years or more. But, with the recent increase in health checkups and guidelines for young workers in their 30s, predicting the onset of MS can be achieved with the collected health data.
In this study, researchers from the University of Yamanashi, Japan, employed machine learning methods, namely random forest (RF) and logistic regression (LR) models, to predict the onset of MS among Japanese workers in their 30s. The study used two sets of 10-year longitudinal health check-up data for 6,048 Japanese employees aged between 30-35 years.
Of the two models investigated, RF demonstrated a higher prediction accuracy compared to LR. The researchers also identified the important predictors of MS risk, and found that they were sex-specific. While diastolic blood pressure was the most important predictor in males, it was the body mass index for females.
Additionally, waist circumference, LDL-C, HDL-C, and skipping breakfast were other important predictors of MS onset in males. In the case of females, uric acid and triglyceride, and restful sleep constituted other important predictors. Moreover, daily exercise habits was a common important predictor for both the sexes.
In summary, the predictive machine learning models developed by the researchers can be used to reliably predict MS onset risks at 40 by analyzing the routine healthcare data of people in their 30s. Moreover, the predictors identified as important could guide appropriate healthcare interventions to mitigate such risks among young workers.
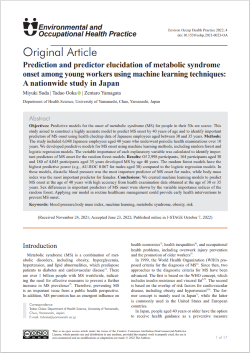
Link to original journal article:
https://www.jstage.jst.go.jp/article/eohp/4/1/4_2021-0023-OA/_article
Title of the paper:
Prediction and predictor elucidation of metabolic syndrome onset among young workers using machine learning techniques: A nationwide study in Japan
Authors:
Miyuki Suda, Tadao Ooka, Zentaro Yamagata